Work on Earth’s Climate and Other Complex Systems Earns Nobel Prize in Physics
Introduction
Physicists have traditionally dealt with simple systems — a single atom, a gas in a box — for which the governing laws are clear and exact answers can be calculated. To describe bigger, slightly more complicated systems, they know how to simplify, stripping things down to their essence and ignoring details that don’t matter. A ball rolls the same way down a ramp whether it’s red or blue.
But in the 20th century, physicists began to grapple with the kinds of complex systems that can’t be stripped down in the usual way. In these systems — such as Earth’s climate — small details matter; they can snowball and effect sweeping changes, in a process called a positive feedback loop. New methods had to be developed to identify the operating principles behind these complex, chaotic systems in order to approximate their behavior. This pioneering work on distilling complexity has been honored by today’s Nobel Prize in Physics.
Syukuro Manabe, a climatologist at Princeton University, and Klaus Hasselmann, at the Max Planck Institute for Meteorology in Hamburg, Germany, received half of the prize for their work in the 1960s and 1970s, respectively, building climate models that have correctly predicted the effects of increasing carbon dioxide in Earth’s atmosphere.
Giorgio Parisi, a physicist at Sapienza University of Rome, has been awarded the other half for discovering hidden patterns in complex physical systems, most notably “spin glasses” — materials made from many simple, interacting parts called spins. Parisi’s studies in the late 1970s and 1980s of how individual, fluctuating spins affect the global properties of the spin glass, and how, in general, the evolution of a complex system as a whole relates to the dynamics of the individual parts that make it up, have allowed researchers to gain a better understanding of a wide range of complex and disordered materials.
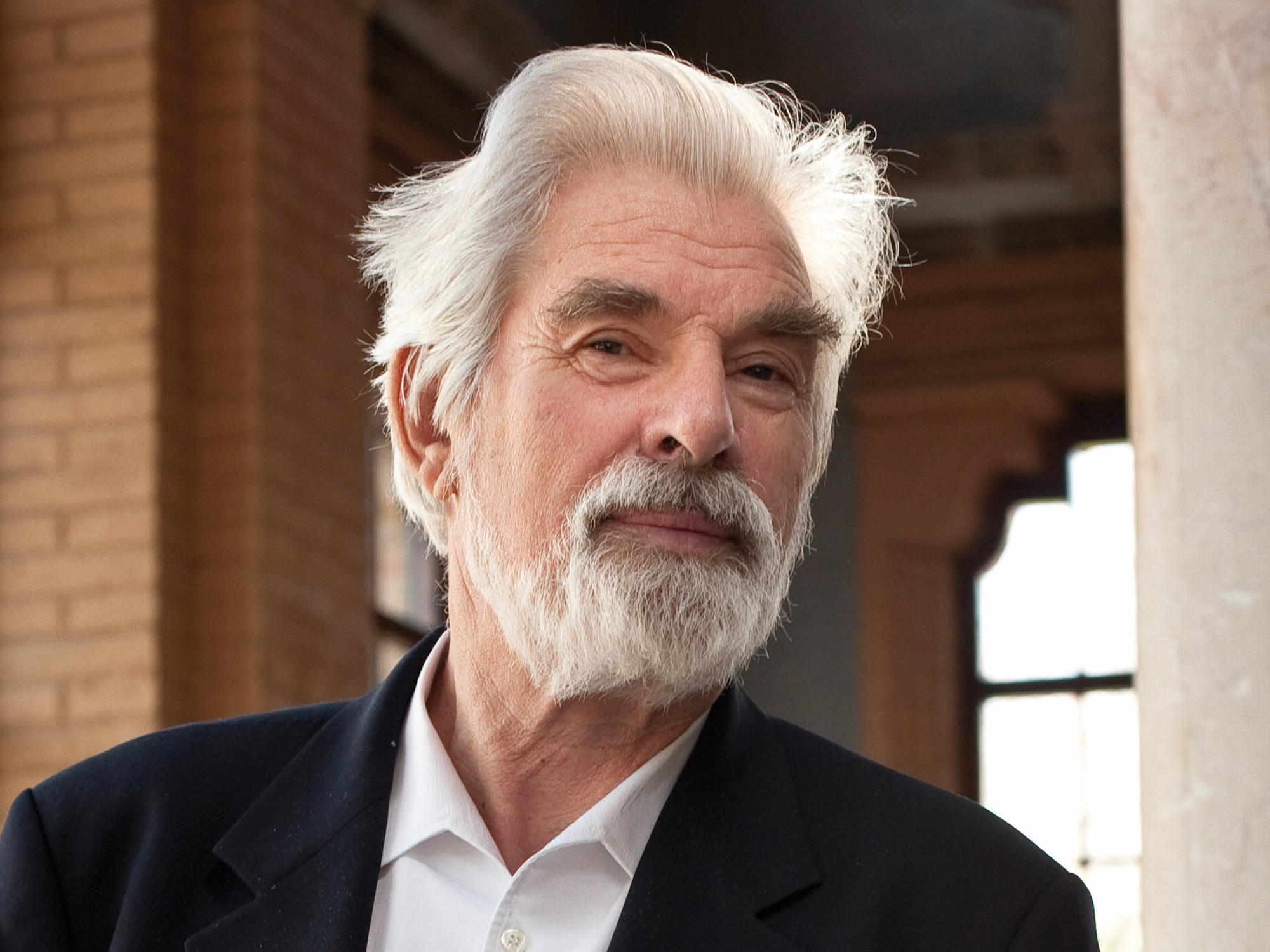
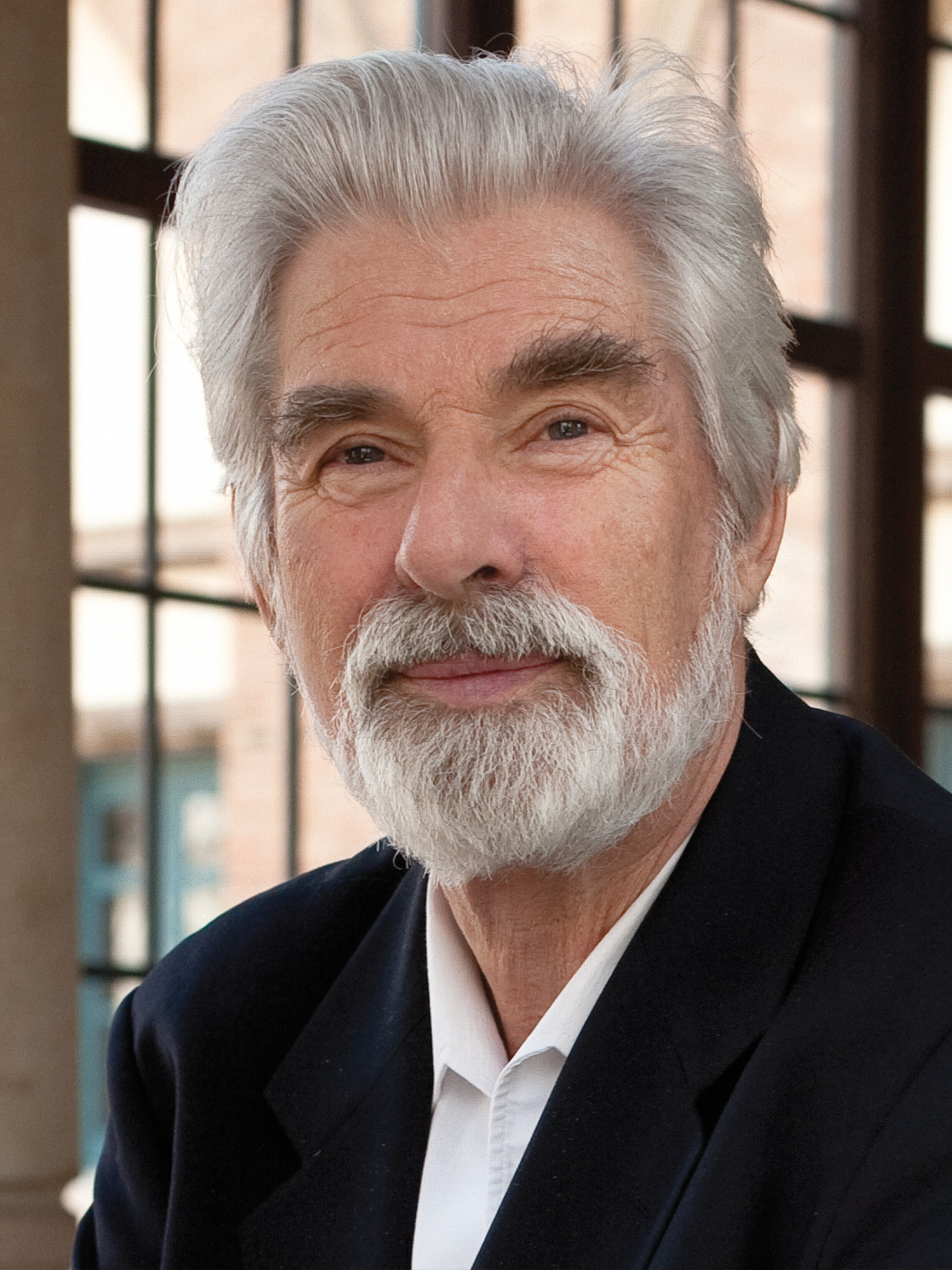
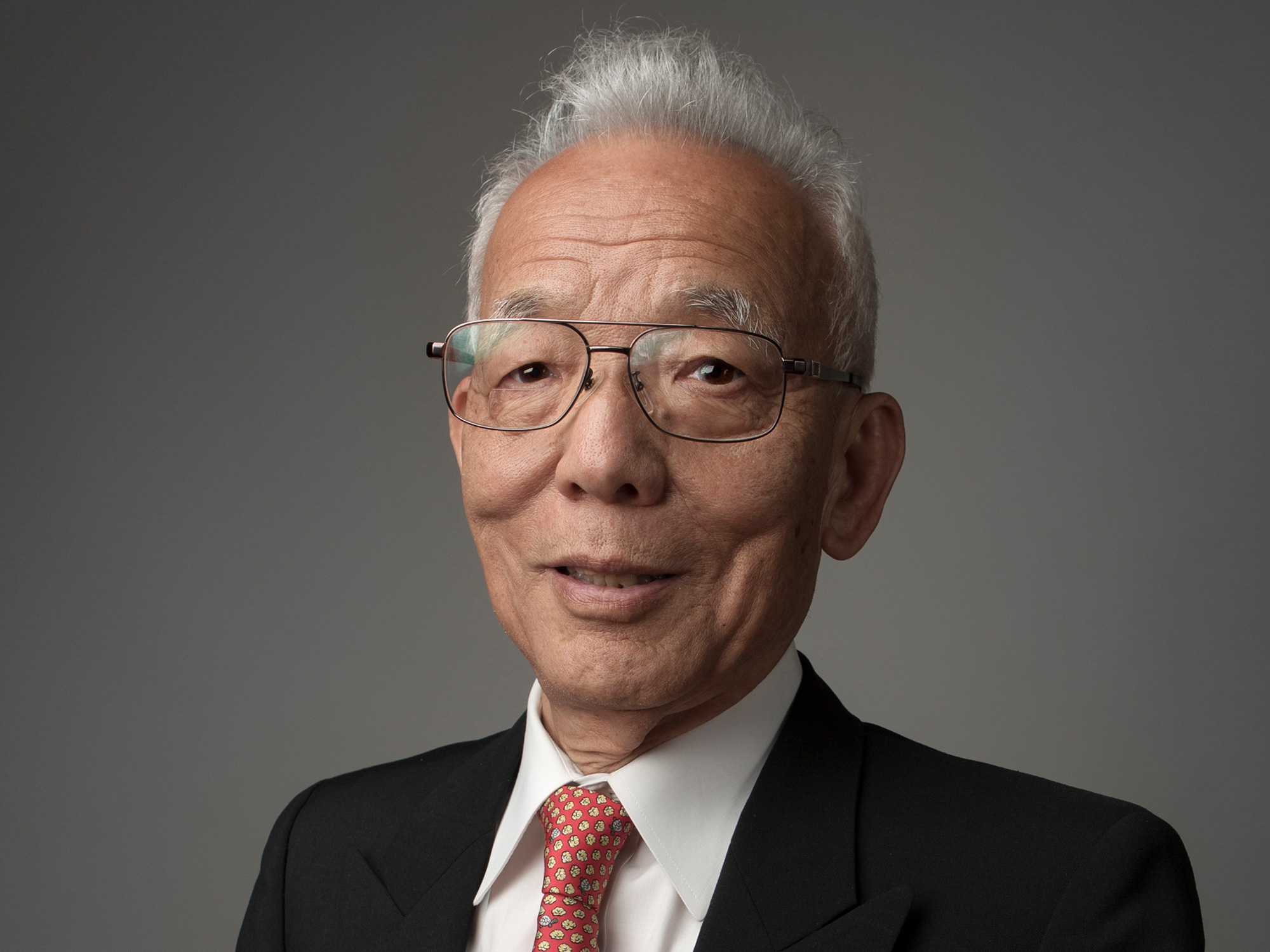
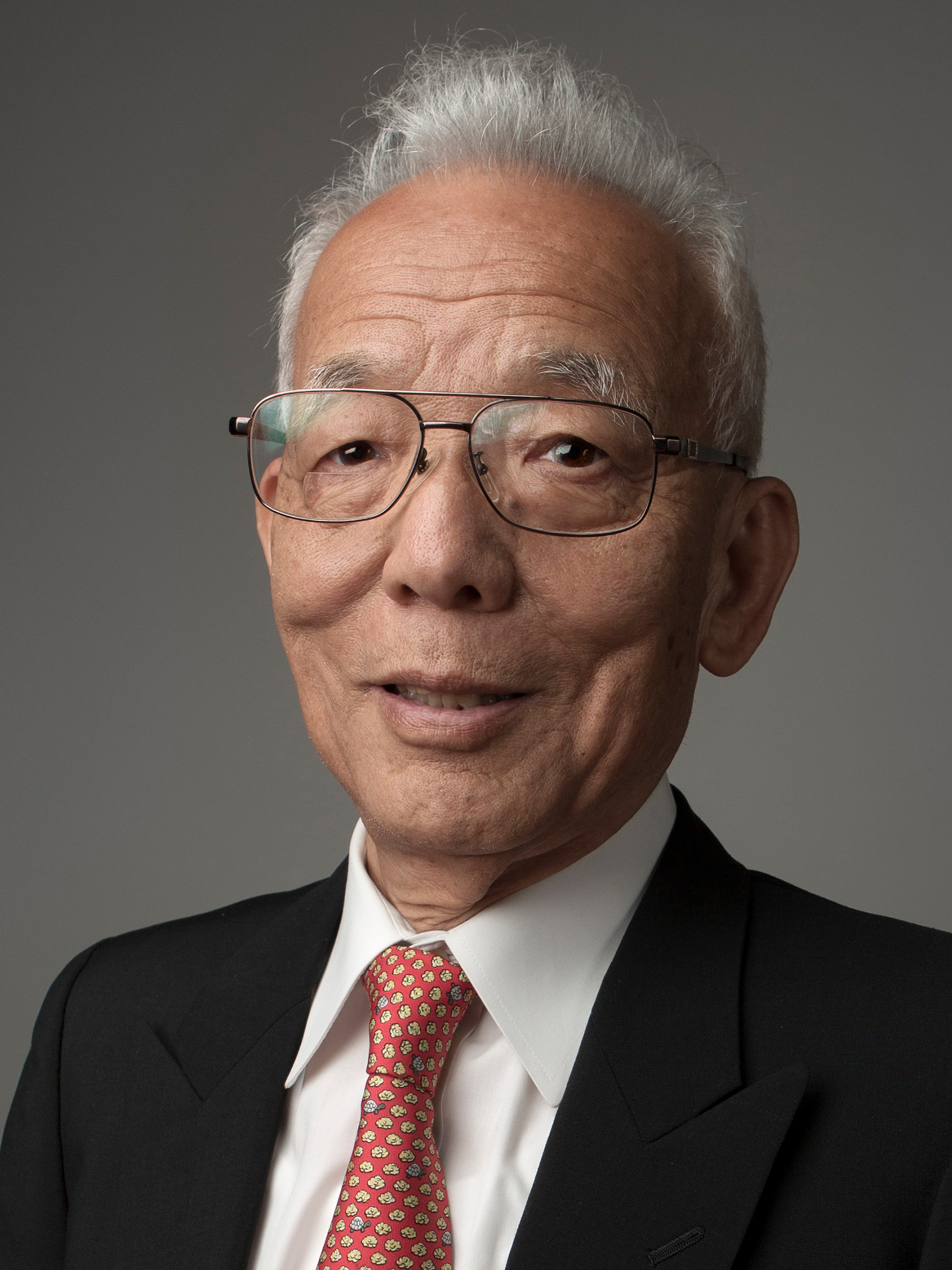
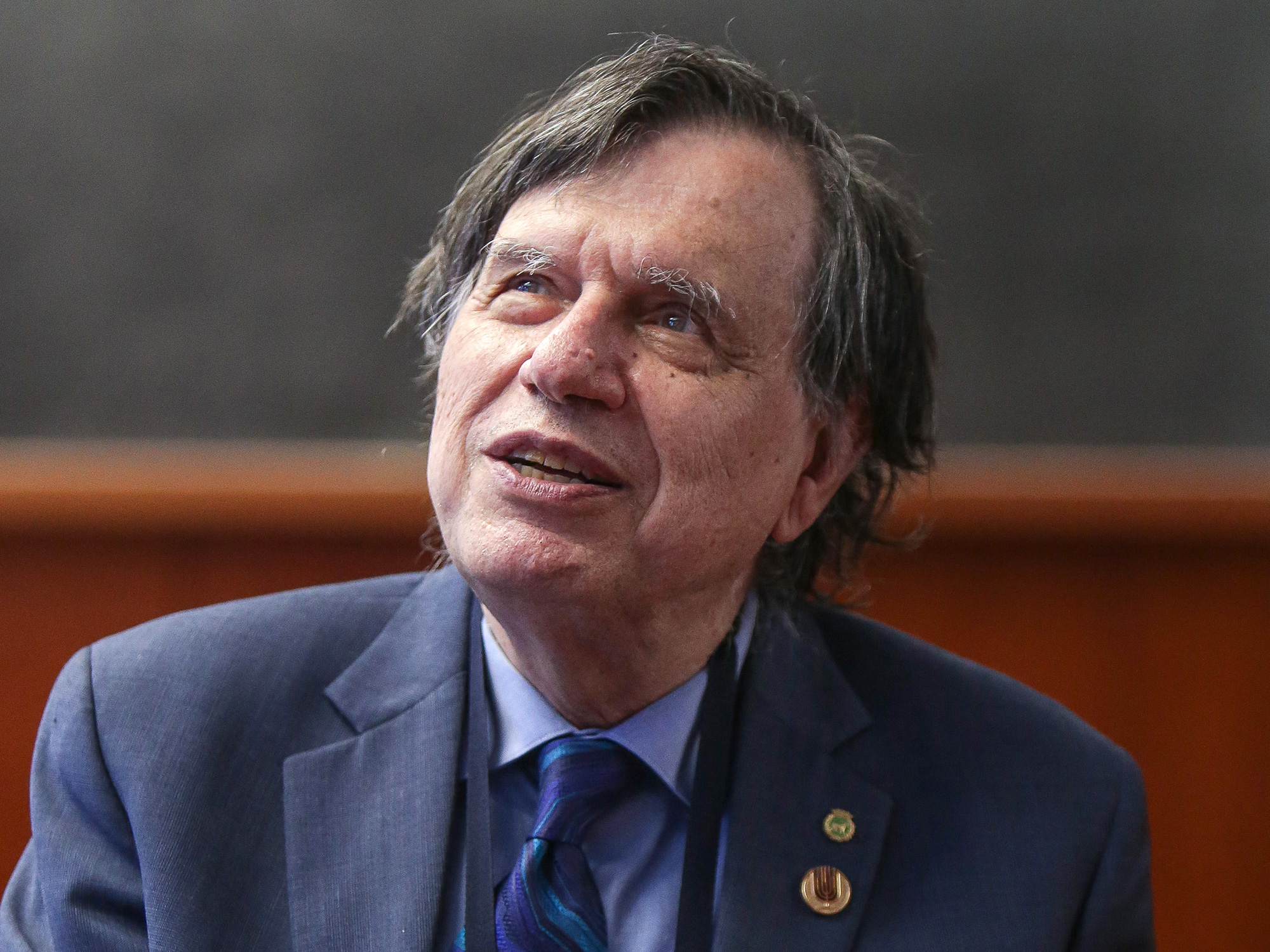
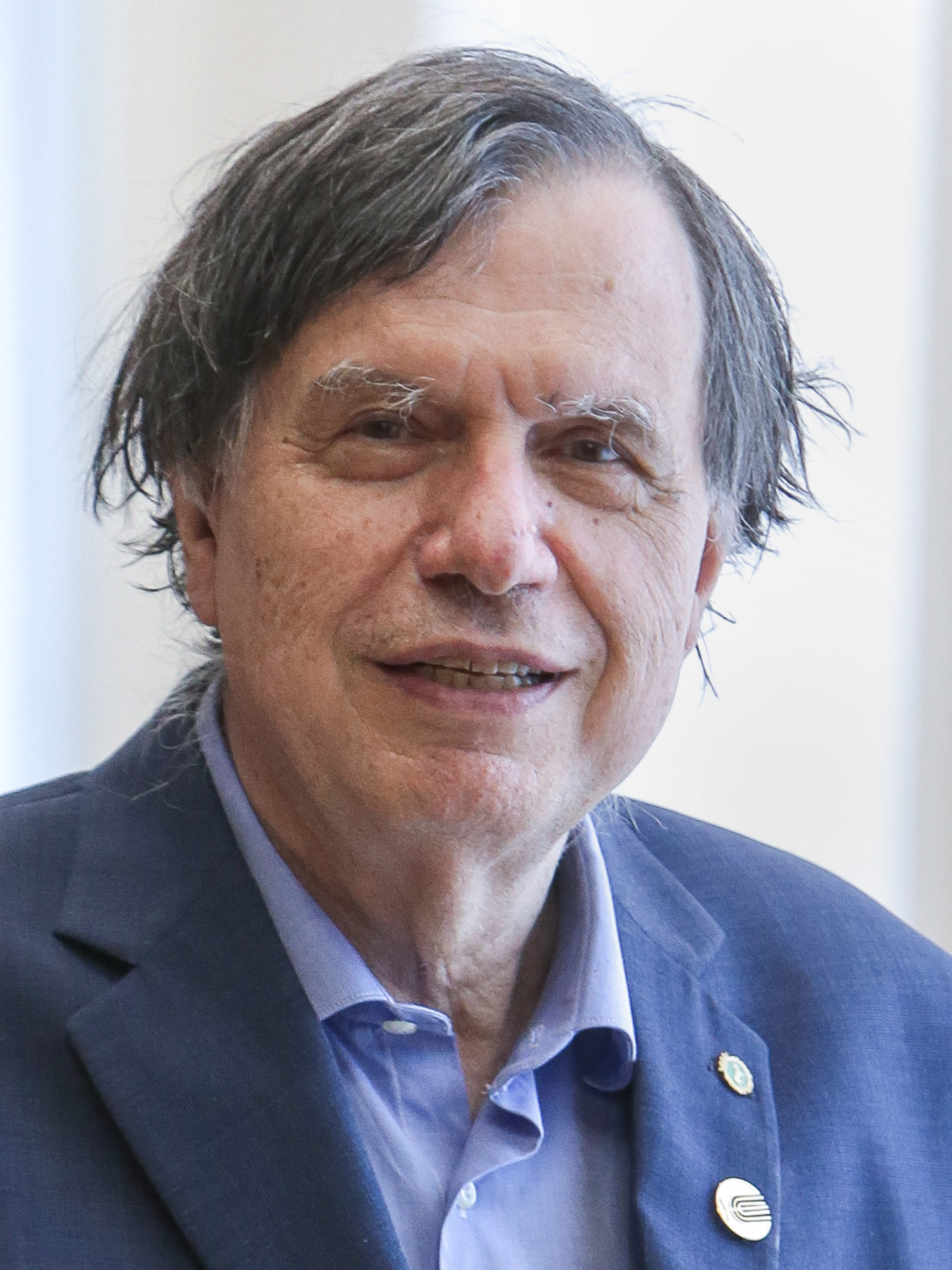
Klaus Hasselmann and Syukuro Manabe (from top) shared half of the Nobel for their work in climate modeling. Giorgio Parisi received the other half of the prize for elucidating a complex system called a spin glass.
Klaus Hasselmann and Syukuro Manabe (from left) shared half of the Nobel for their work in climate modeling. Giorgio Parisi (right) received the other half of the prize for elucidating a complex system called a spin glass.
BBVA Foundation Frontiers of Knowledge Awards; Markus Marcetic, the Royal Swedish Academy of Sciences; Wolf Foundation
All the work of the laureates carries a stark message, which Parisi articulated in answer to a question from a reporter at this morning’s award ceremony in Stockholm. “It is urgent that we make real, very strong decisions, and move at a very strong pace” to address climate change, Parisi said, “because we are in a situation where we can have positive feedback and that may accelerate the increase of temperature. It is clear that for future generations we have to act now in a very fast way, and not delay.”
What is a climate model?
Physicists since Svante Arrhenius in 1896 have strived to strip down Earth’s climate to its essence. They approximate reality by identifying the most important forces at play within a system — those that have a large overall effect on its dynamics. They then work up interlinked equations that describe those forces, while ignoring less significant details in order to make the models solvable.
But the climate is a nonlinear system, where changes in one variable can touch off another, leading to unstable feedback loops. This makes it difficult to identify which small effects can be disregarded. Arrhenius identified water vapor as one such problem area: When the temperature of the air increases, so does its water vapor content, which causes it to trap more of Earth’s heat below, leading to a further rise in temperature. Ice loss is another cause of feedback: Ice reflects sunlight, so when there’s less of it, more sunlight is absorbed by the dark ocean, causing warming that further accelerates ice loss.
Modern climate models take into account the complicated interaction and feedback between millions of variables. They do this by solving a system of equations representing the conservation of mass, momentum and energy at every point on a 3D grid representing the atmosphere of the Earth. The equations attempt to capture the effects of everything smaller than the grid, such as individual clouds or land features. Sometimes these tiny features can have large effects that aren’t captured by the model, which means that the finer the grid, the better the model. But this accuracy comes at the cost of formidable computing resources. A state-of-the-art model might require weeks or months of processing time on the world’s fastest supercomputer, which can execute 1.5 quintillion computations per second.
When climate modelers were first getting started, they had to consider a much smaller, bite-size piece of the climate puzzle.
What did Manabe’s early climate models show?
Manabe’s models in the 1960s “can be considered as the first realizations of the dream of Arrhenius,” according to the Nobel committee. These models considered a single, vertical column of the atmosphere stretching from the ground to the stratosphere. Treating this column as a series of grid points, Manabe and his collaborators studied how the concentration of a greenhouse gas like carbon dioxide within the column affects the flow of heat and air between grid points.
He accounted for the fact that hot air is lighter than cold air, so it rises, and that hotter air also carries more water vapor, which is a powerful greenhouse gas. (Greenhouse gases such as water vapor and carbon dioxide strongly absorb and reemit the heat radiating upward from Earth’s surface, blocking much of it from escaping to space. This is the key mechanism that causes warming.) Manabe and his collaborators also considered that, high up in the column, the air is colder, and so cloud drops form, releasing latent heat stored in the water vapor.
By accounting for the interplay between these variables in each column of the sky while ignoring horizontal transport of air and heat, they could extrapolate to the whole globe and approximate the overall “sensitivity” of the climate: how much the air temperature increases as a result of doubling the carbon dioxide level. Their early estimates — that the global temperature would rise by 2.3 degrees Celsius in response to doubling of the carbon dioxide concentration — remain impressively accurate even when measured against modern climate models.
By 1975, Manabe and his colleague Richard Wetherald had extended their column model into a primitive global model, calculating (on a computer with half a megabyte of RAM) the global climate sensitivity to be 2.93 degrees Celsius. Current models predict a range of 2.5 to 4 degrees Celsius for the climate sensitivity. To gauge the extent of this predicted level of warming, consider that Earth during the last ice age was 6 degrees Celsius colder than it is today.
How does weather interact with climate?
Climate is long-term; weather is short-term. And yet the two are related. How?
In the 1970s, Klaus Hasselmann developed climate models that incorporated weather events, instead of averaging over them, as had been done before. He relied on the premise that the climate slowly evolves as an overall response to the fast, random fluctuations we know as weather. To do this, he developed equations that coupled a slowly varying function representing the climate with a rapidly varying function representing weather. He showed that the latter impacts the former in the same way that the random motion of air molecules on microscopic scales affects the random motion of dust particles on macroscopic scales — an effect called Brownian motion. This analogy with Brownian motion proved very powerful as a way of understanding the interplay between different physical scales in the climate system.
How do climate modelers distinguish natural and human causes of climate change?
For decades, researchers struggled to systematically tease apart the effects of random weather events, volcanic eruptions and other natural changes from human-caused climate change. In three papers spanning from 1979 to 1997, Hasselmann created a framework for doing this, a way of comparing climate models and observations in order to assess whether the models were faithfully capturing the effects of natural variability.
In his framework, he devised “optimal detection” techniques for identifying the signals of human-caused warming. He showed that such signals are best detected, for instance, not in the parts of climate data where they are strongest, but where noise is weakest. A recent article about the history of climate science described one of Hasselmann’s optimal-detection papers as “the first serious effort to provide a sound statistical framework for identifying a human-caused warming signal.” The authors go on to write that “Instead of looking for a needle in a tiny corner of a large haystack (and then proceeding to search the next tiny corner), Hasselmann advocated for a more efficient strategy — searching the entire haystack simultaneously” for signals with unique characteristics, or “fingerprints,” that show they do not stem from noise.
The fingerprints of human-caused warming grew more pronounced as the decades wore on, driving a growing consensus among climate researchers. Earlier this year, the Intergovernmental Panel on Climate Change concluded that “it is unequivocal that human influence has warmed the atmosphere, ocean and land.”
What is a spin glass?
As researchers like Manabe and Hasselmann grappled with the climate, Giorgio Parisi’s muse from the late 1970s on was a physical system called a spin glass. This is a material containing magnetic particles — iron atoms, for instance — which you can think of as tiny bar magnets pointing either up or down. In work that strongly resonated with Hasselmann’s insights about weather and climate, Parisi studied how the back-and-forth flipping of spins affected the slow dynamics of the spin glass as a whole.
Spin glasses have captivated physicists like Parisi because of a phenomenon known as “frustration.” Imagine a simple spin glass consisting of three spins at corners of a triangle. Adjacent spins prefer to point in opposite directions. But the three spins, as they flip back and forth trying to attain a stable configuration, can’t all satisfy that constraint simultaneously; the system is what physicists call “frustrated.” Frustration means that spin glasses must compromise; they seek the least bad configuration. Parisi’s studies of how this plays out in spin glasses “were so deep,” according to the Nobel committee, “that they not only influenced physics, but also mathematics, biology, neuroscience and machine learning, because all these fields include problems that are directly related to frustration.”
Editor’s Note: Giorgio Parisi has received funding from the Simons Foundation, which also funds this editorially independent magazine. Simons Foundation funding decisions have no influence on our coverage.
This post has been updated with additional details about the award-winning work.
David Julius and Ardem Patapoutian won the 2021 Nobel Prize in Physiology or Medicine. Benjamin List and David MacMillan won the 2021 Nobel Prize in Chemistry.