Chasing the Elusive Numbers That Define Epidemics
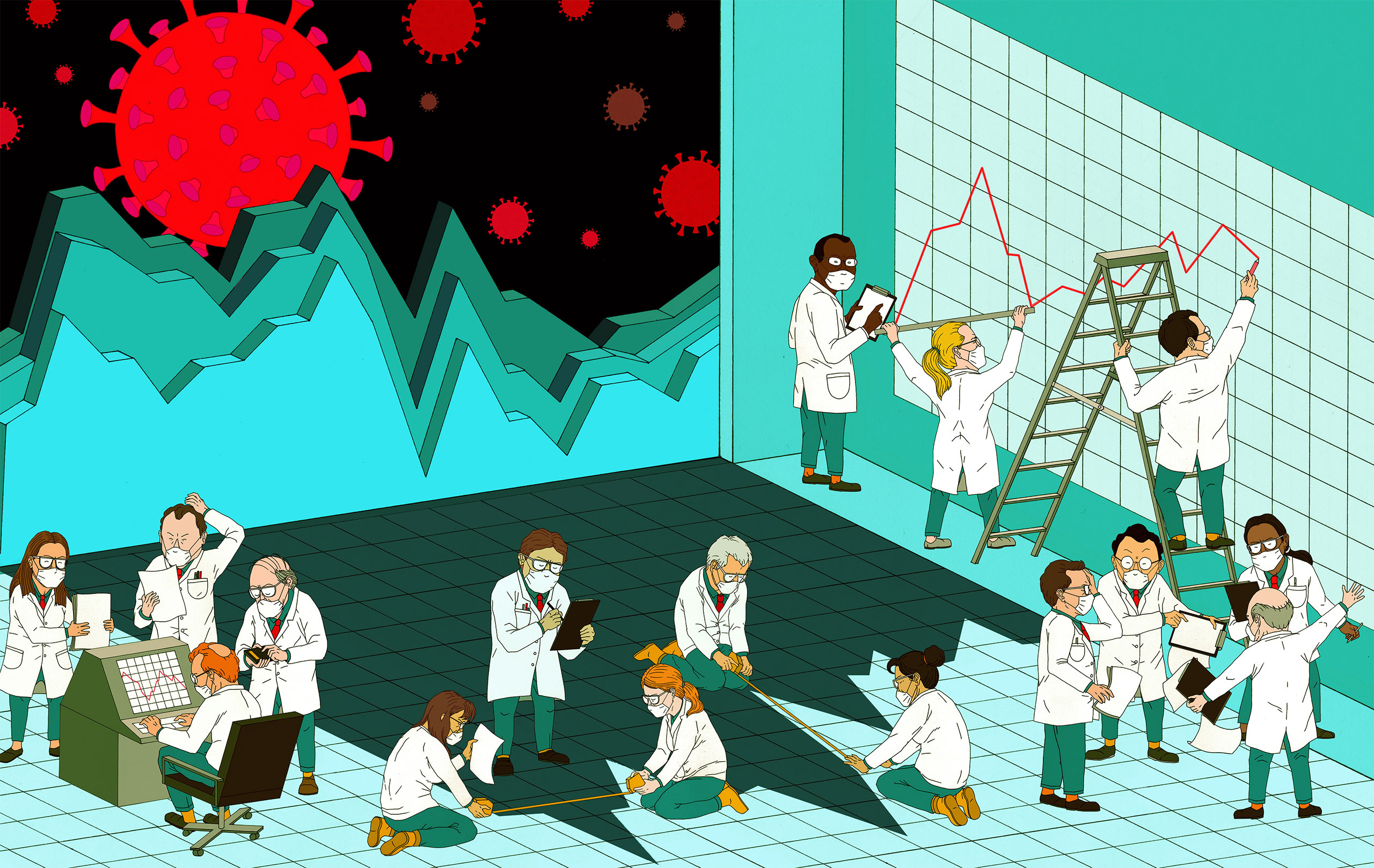
Researchers can’t directly observe many key features of disease transmission. As a result, they rely on statistical models to translate what they can see to what they want to know. But they’re finding that for COVID-19 in particular, some of these methods have been giving them the wrong answers.
Jon Fox for Quanta Magazine
Introduction
Variables in epidemiological models aren’t usually well known to the general public, but one has had a genuine movie star moment. “What we need to determine is this,” says a scientist played by Kate Winslet in the film Contagion. “For every person who gets sick, how many other people are they likely to infect?” On a whiteboard, she writes down the answer for several familiar diseases: around 1 for seasonal flu, upward of 3 for smallpox, between 4 and 6 for polio.
That value is the basic reproduction number, R0 (pronounced “R-naught”) — the average number of infections that one case generates in a completely susceptible population. When an epidemic emerges, this is what everybody immediately scrambles to estimate, because it can signal how aggressively a novel pathogen might spread, how large an outbreak could become if left unmitigated, and the threshold at which herd immunity might be reached. It can help to gauge how difficult it might be to control the disease, and how to go about doing so.
But assessing a disease’s transmission parameters can be immensely difficult and subject to pitfalls that even the experts don’t always foresee: Throughout the COVID-19 pandemic, for instance, estimates of R0 have ranged widely, from under 2 to between 6 and 7.
And so, while much of the modeling work over the past year has focused on addressing the world’s most urgent and practical questions about COVID-19, some research teams have instead delved deeper into the underlying theoretical concerns. They’ve sought to gain new, fundamental insights into parameters like R0 — into what those variables really mean, how to estimate them, and when they should or shouldn’t be used.
Those scientists aim to lay important groundwork for the inevitable next pandemic. “I feel it’s worth understanding,” said Jonathan Dushoff, a theoretical biologist at McMaster University in Canada. The hope is that “we’ll have more solid tools, so that by the time the next epidemic comes along, this stuff will be one less thing to worry about.”
The Speed and Strength of Epidemics
The fundamental hurdle is that R0 can’t be measured directly. If epidemiologists were all-seeing, they could learn R0 by counting the number of cases that each infected person causes and taking the average. But in practice, they can’t observe those infection events, leaving them to estimate R0 from statistical models based on observed data.
To bridge the gap between what’s observable — the rate at which the epidemic is growing or shrinking, or what Dushoff calls its “speed” — and the desired value R0, or “strength,” another important quantity is needed. That’s the generation interval: the amount of time between when one person is infected and when they infect the next person. (Since that value can vary greatly, researchers might represent the generation interval as a single number, like the mean, or as a distribution.)
“There’s often a conflation,” said Joshua Weitz, a biologist at the Georgia Institute of Technology, in thinking “a faster growth rate must mean a higher R0.” But that growth rate really needs to be viewed through the lens of generation intervals, and how quickly one infection leads to another.
Consider a situation in which one initial case of a disease is followed, three weeks later, by the appearance of eight new cases. If the disease has a generation interval of one week, then that initial case would have led to two new cases after the first week, four the next, then eight. Each infection would have produced two others, for an R0 of 2. But if the disease instead has a generation interval of three weeks, then the first case directly produced the eight new ones, for an R0 of 8.
Samuel Velasco/Quanta Magazine
“We don’t have a one-to-one relationship between what we observe and what we would like to know,” Weitz said. That the same observed case count can be explained by very disparate values of R0 is a challenge “that is still not as well appreciated in the field.”
Weitz and Dushoff came face to face with that fact during the 2014-2016 Ebola epidemic in West Africa. They realized that if postmortem transmission — from the handling of the deceased during funerals — was a primary source of new Ebola infections, then most experts were probably assuming generation intervals for the disease that were too short. That meant published R0 values were probably underestimates, and medical authorities could be embracing the wrong priorities to stop the outbreak. Indeed, researchers would later confirm the importance of postmortem infections in Ebola’s spread.
Estimating R0 for COVID-19
With that experience in mind, Dushoff and Weitz, along with Sang Woo Park, a graduate student in ecology and evolutionary biology at Princeton University, set out last year to make sense of the divergent R0 estimates for COVID-19. As soon as Weitz saw the extent to which those estimates varied, “it smelled to me like what they were really doing was making a different assumption about generation intervals,” he said.
When he, Dushoff and Park combed through different groups’ calculations, that’s exactly what they found. They also showed that if researchers only took into account the transmission dynamics of symptomatic individuals, they would likely miscalculate R0. Some studies have found that asymptomatic individuals spread the virus for longer, both because they may have a prolonged viral shedding period, and because they are more likely to avoid detection and continue transmitting the disease.
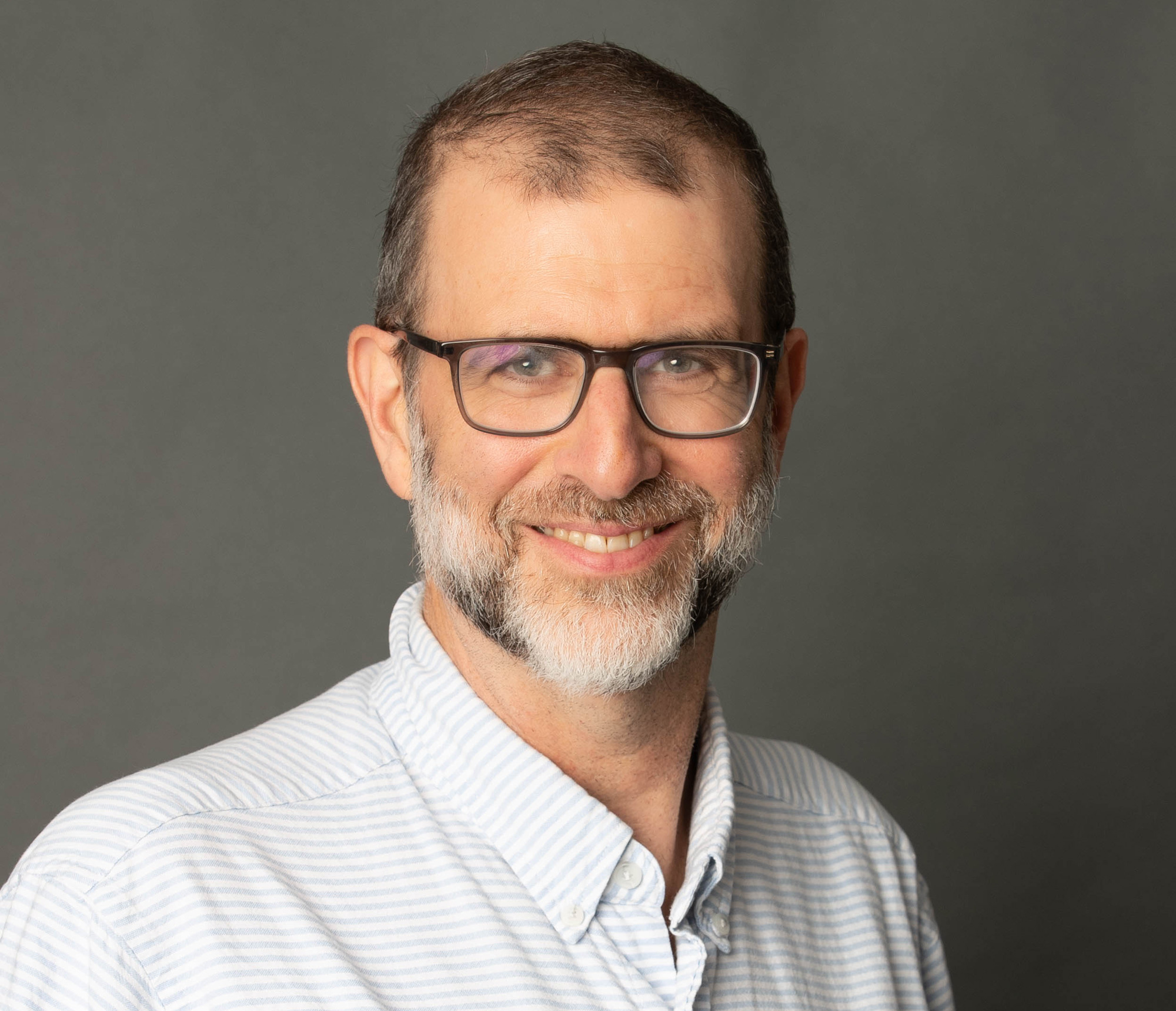
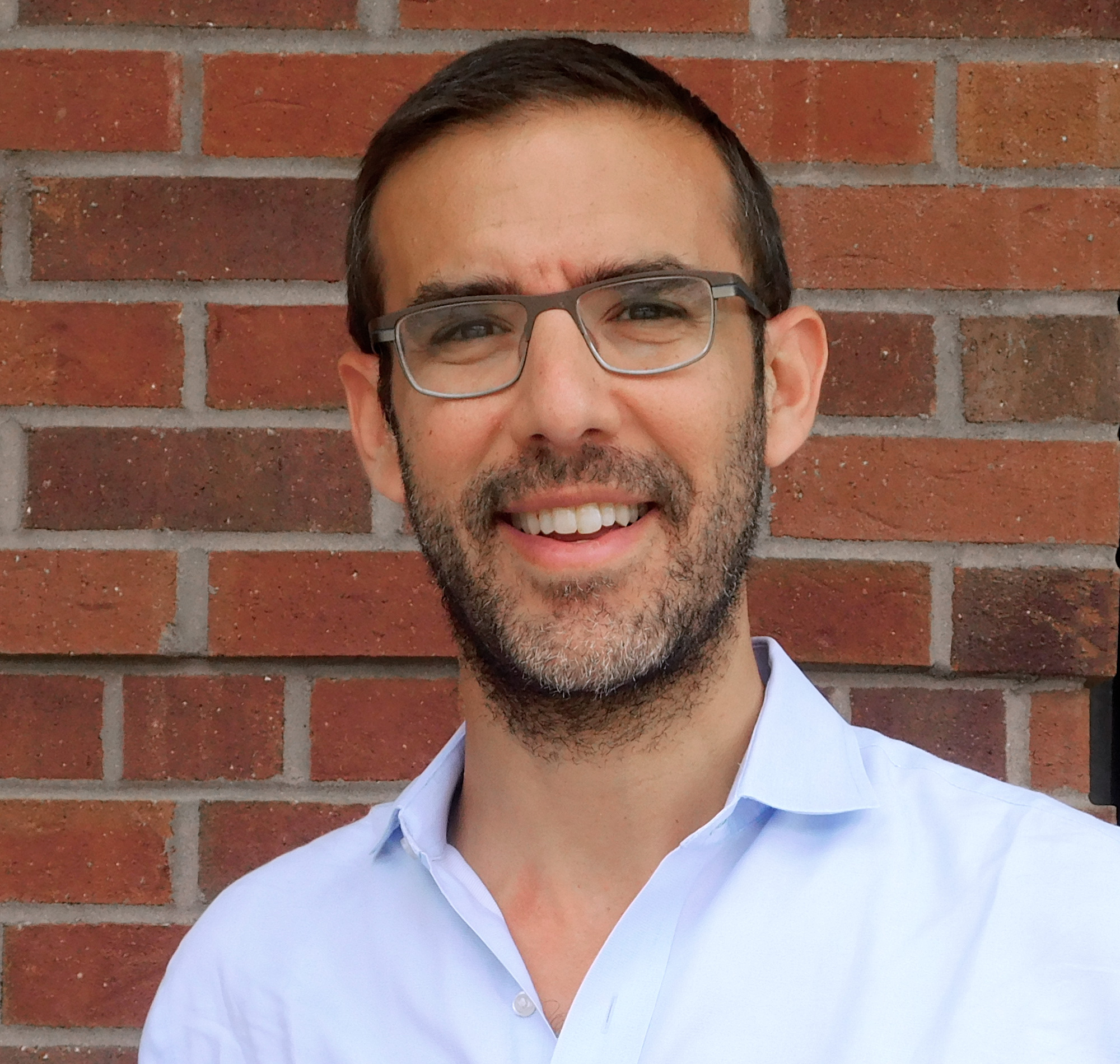
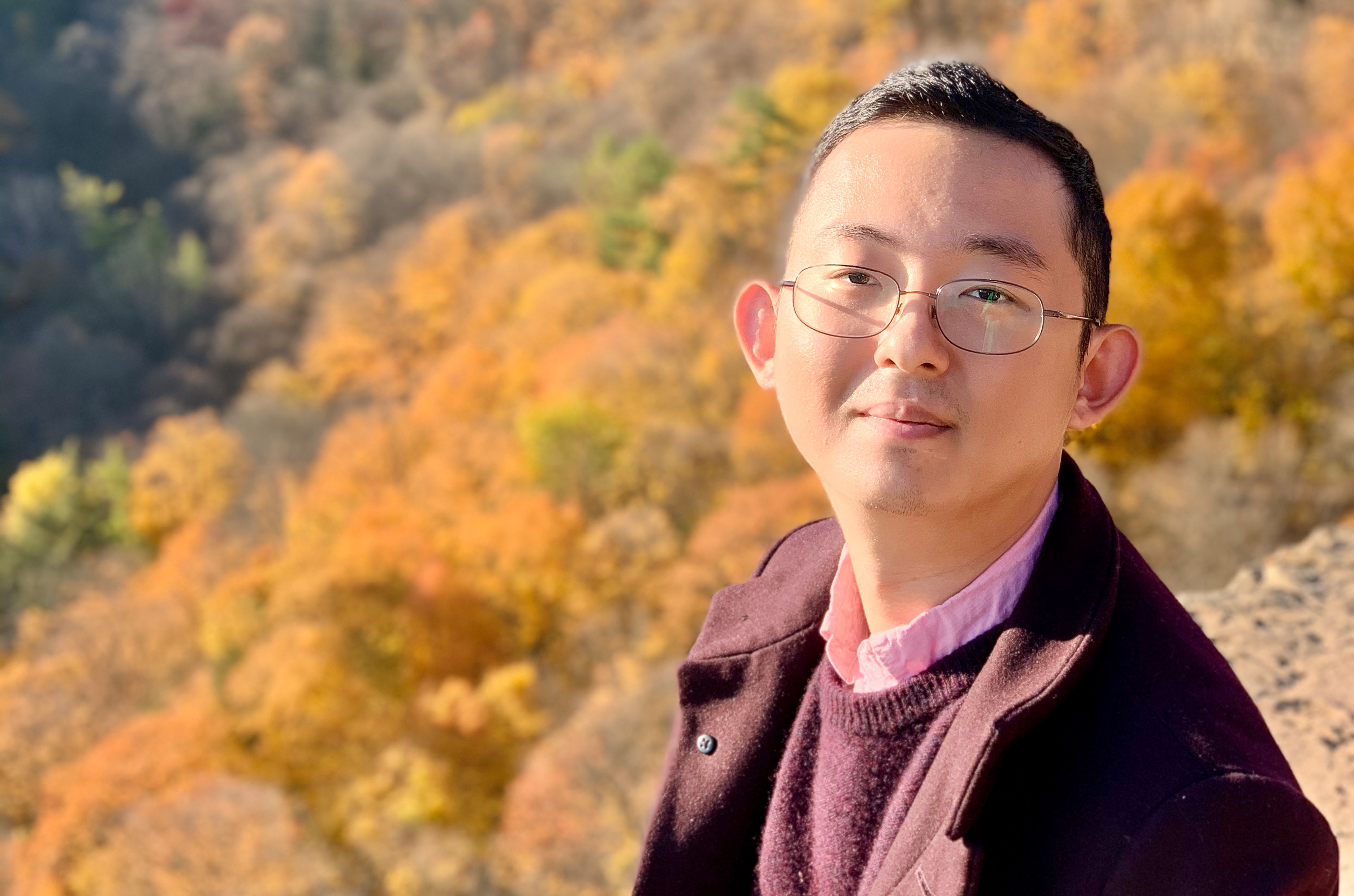
Biologists Jonathan Dushoff, Joshua Weitz and Sang Woo Park have been developing a better understanding of the theory behind the basic reproduction number, R0, and how to estimate it for diseases like COVID-19.
McMaster University; Georgia Tech; Courtesy of Sang Woo Park
If symptomatic and asymptomatic transmission “have different generation intervals, then that’s going to fundamentally change our estimates, and therefore change our understanding of current risk and [future] scenarios,” Weitz said.
Weitz added that these findings underscore the importance of settling on a firm definition of asymptomatic spread and determining whether its rate changes over time or in different populations. “These things are going to lead to very different kinds of outcomes” and responses, he said (such as the prioritization of rapid mass testing for COVID-19).
Another consideration is that the generation interval for COVID-19 has probably decreased over time. Even when researchers first began calculating R0, interventions such as lockdowns and test-trace-isolate efforts were already reducing people’s contacts and cutting transmission periods short. But estimates of R0 need to be based on the unmitigated epidemic, so if generation intervals are inferred after some of these changes have occurred, scientists once again risk underestimating R0.
This work has led Weitz and others to reinterpret some aspects of the disease’s propagation. Over the summer, for instance, “there was a narrative that cases were spreading in young people, and that their [irresponsible] behavior was driving that spread,” Weitz said. But behavioral factors alone might not be to blame: If younger people were biologically more likely to transmit the virus asymptomatically, they might have had an outsize impact on the rate of spread, simply because asymptomatic transmission has a longer generation interval. Weitz notes that the results are still preliminary and incomplete, but he thinks they are “intriguing” and might help us “begin to walk away from this notion of culpability” where it isn’t appropriate.
Toward Getting It Right
Part of what makes ascertaining the generation interval of COVID-19 so complicated is that, like R0, it can’t be directly observed, because the timing of infections is often unknowable. Researchers must turn to a proxy instead — the serial interval, the average time that elapses between when someone first develops symptoms and when a person they infect does.
But serial interval values are usually obtained from careful contact tracing and related epidemiological studies, neither of which is available early in an epidemic. This leads to different assumptions and a great deal of uncertainty about what the interval might be.
And although the generation and serial intervals are conceptually similar, they are fundamentally different. For example, the generation interval is always a positive value. But in diseases like COVID-19 that involve large amounts of presymptomatic transmission, a patient sometimes develops symptoms before the person who infected them, so the serial interval can be negative. (And in cases of asymptomatic transmission, the serial interval is undefinable.) Park says that the SARS-CoV-2 virus was what made him realize “they needed to develop a better framework for capturing” the complexity of transmission dynamics.
Samuel Velasco/Quanta Magazine
Moreover, the researchers uncovered yet another statistical difficulty: It mattered greatly how individuals were grouped and how their transmission intervals were measured. Estimates of serial interval from contact tracing data usually work backward, from a starter group of infected individuals to the people who infected them. But that approach turns out to be more vulnerable to statistical bias than measuring the serial interval forward, from the starter group to the people they infect. To address that, Dushoff, Park, Weitz and their colleagues are digging deeper into how to use the appropriate reference points to get more accurate estimates of R0.
“We aren’t done yet,” Dushoff said. This is something they, and the rest of their field, still need to grapple with. But they’re starting to disentangle the issues one by one — looking at individual timescales of transmission, and the perspectives they’re viewed from, to pin down just how important they are for understanding the dynamics of a disease.
Shifting to Rt
Although a good estimate of R0 is in high demand at the beginning of an epidemic, its immediate usefulness diminishes as the months drag on. Interventions taken to curb transmission, the rise of immunity among people who recover, and other factors change the reproduction number for a disease over time. As an epidemic progresses, researchers gradually shift their attention from R0 to that real-time value, known as Rt.
Like R0, Rt is often derived from serial intervals and inferred generation intervals — and because those intervals also evolve throughout the epidemic, related challenges apply. But Rt tends to be most sensitive to assumptions about serial and generation intervals when the epidemic’s exponential growth is relatively high (often the time when R0 is more relevant). As a result, some of the uncertainties affecting R0 start to matter less with Rt.
Better still, at least in principle, Rt can serve as a real-time indicator of the epidemic’s potential for spread, and of how well interventions are working. If Rt is greater than 1, the epidemic is growing, and more mitigation measures may be needed. If it’s less than 1, the epidemic is shrinking, and policy setters might consider lifting some restrictions.
The danger, however, is that Rt is still difficult to evaluate accurately. And if Rt is significantly underestimated, decision-makers may think they have more headroom to relax interventions than they actually do.
Those concerns loomed large for Sarah Cobey, an ecologist at the University of Chicago, when she came across a paper in JAMA last April that sought to estimate changes in Rt over the early course of the epidemic in Wuhan, and to overlay those estimates with the timing of different policies. One implication of the paper’s analysis was that Rt didn’t dip below 1 in Wuhan until the Chinese government started enforcing centralized quarantining.
But Cobey, along with the Harvard University epidemiologists Marc Lipsitch and Keya Joshi, expressed concerns about using Rt to make such a claim. They pointed out that different methods can estimate values of Rt that are slightly offset from each other in time. “If Rt had actually fallen below 1 just four or five days earlier, they would have inferred something totally different about what level of intervention was necessary to cause the epidemic to start contracting,” said Katelyn Gostic, a postdoctoral researcher in Cobey’s lab.
Samuel Velasco/Quanta Magazine; source: Marc Lipsitch et al.
To make Rt estimates that are temporally accurate, researchers need to infer when infections occur based on when cases, hospitalizations or deaths are reported. However, the delays between the time when people get infected with COVID-19 and when they’re observed as a case (or when they are hospitalized or die) make that almost prohibitively difficult.
Gostic and others found that established statistical techniques for dealing with those delays weren’t working well for the COVID-19 pandemic. They tested a variety of published methods for estimating Rt on simulated data, knowing what the underlying Rt values and their time stamps should be. Even then, they didn’t always get the right answers. “It turned into a whole bunch of back-and-forth,” Gostic said, “of us trying to figure out if we weren’t getting the right answers because we had made a mistake, or because the methods were just fundamentally not going to give back the right answer.”
It turned out to be the latter. “The tools that we had ready to go before the pandemic had sort of missed a lot of details that we suddenly had realized were important,” Gostic said — particularly the effects of delays in reporting. “And so, as an epidemiologist, you get back these noisy data streams that we know are lagging indicators of actual changes in the epidemic. And then it’s up to us to try to figure out how to adjust them properly.”
To do that, she and others have turned to methods often used in signal or image processing. They’re also building on statistical approaches used in the 1980s and ’90s during the HIV/AIDS epidemic, which was characterized by even longer delays between infection and case observation.
The researchers accept that there will always be some uncertainty surrounding Rt estimates. Even so, Gostic hopes that her team’s work, as well as other efforts, will be useful going forward — “that in future pandemics, we can just pull this off the shelf and have it ready to go, in a way that we didn’t really this time around.”
A More Complete Picture
The quest to pin down good estimates of R0 and Rt also demonstrates that these parameters aren’t sufficient or reliable enough to provide a rich understanding of an epidemic — as might be expected. “When I think about today’s weather, I don’t think we’re satisfied as a society with saying one number, the temperature,” Weitz said.
Scientists are therefore looking for other numbers to characterize the epidemic. Some have prioritized a parameter that reflects the heterogeneity and variance involved in disease transmission. Others, like the computer scientist Zachary Lipton and his team at Carnegie Mellon University, have been developing novel data signals that go beyond the numbers of cases, hospitalizations and deaths “to view this monster from a different angle,” he said. Those new signals include the fraction of people who have recently observed someone with COVID-like symptoms, the fraction of doctors’ visits for such symptoms, and dozens of other indicators.
Weitz built a risk calculator to determine whether one or more people at events of different sizes in different places might have COVID-19. “One of the challenges has been … what does one do, as a layperson, with even Rt?” Weitz said. “But people understand what it means to think about going to a 50-person event and being told there’s a 25% chance you may be exposed to COVID-19.”
Applications aside, Weitz considers the more theoretical studies he and others are doing on R0 and Rt to be crucial. “Sometimes you need to do some of the foundational work,” he said. “Otherwise, you’re not going to have the basic research to make the generalizable finding.”
Dushoff agreed, adding, “We need more detailed and realistic models, absolutely.” But he thinks the models will probably be more successful when they are guided by an intuitive understanding of how the viruses spread. “And I think we have more intuitive understanding to build,” he said.