Why Don’t Patients Get Sick in Sync? Modelers Find Statistical Clues.
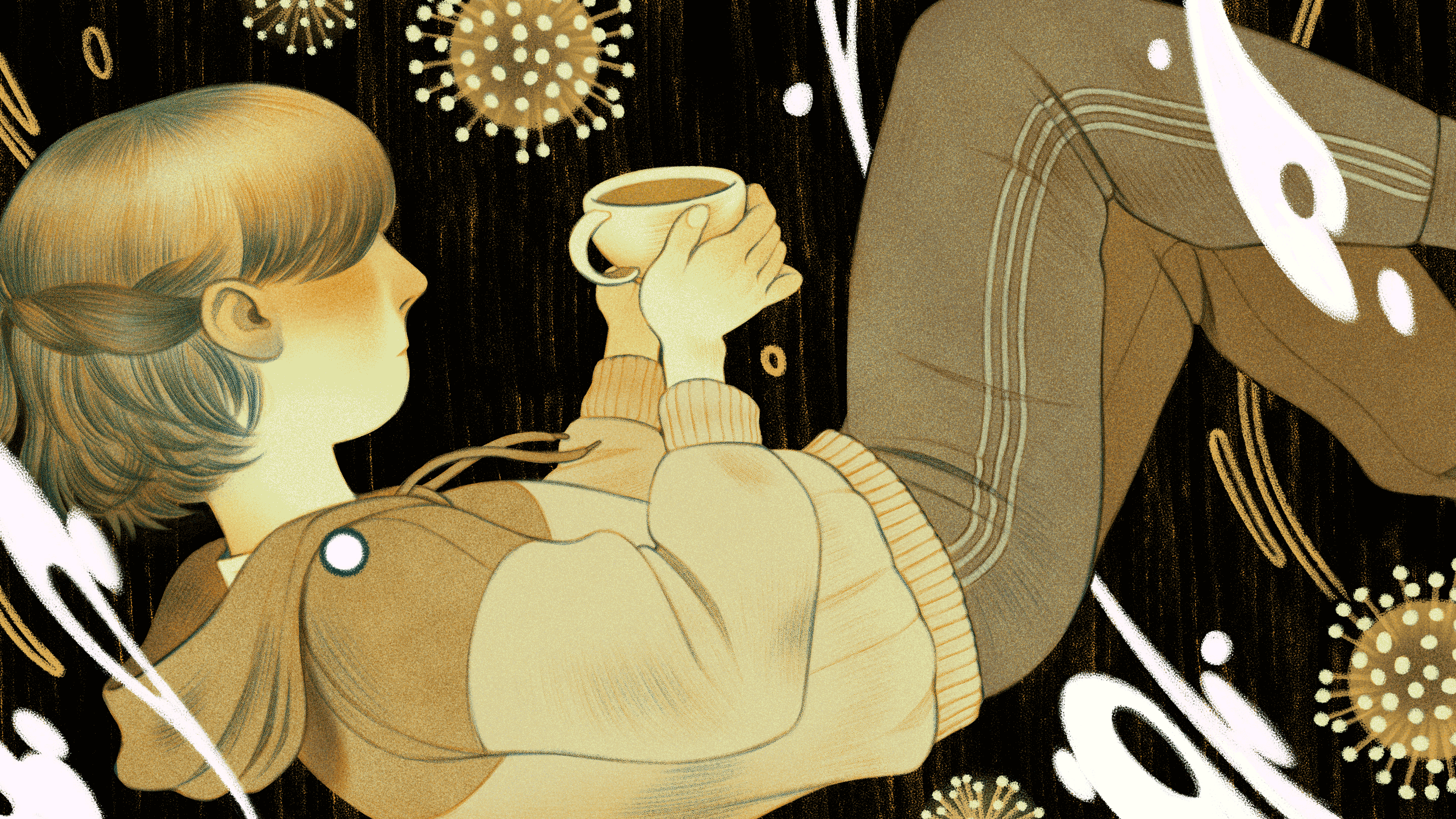
Why do some people get sick after exposure to a disease sooner than others? The answer might reflect statistical principles for cells spreading through tissues.
Rachel Suggs for Quanta Magazine
Introduction
In late March of 1914, the town of Hanford, California, was rocked by contagion. First, a few people got sick. Then over the next month, nearly a hundred more followed, vomiting and shivering with fever, and blood tests made it clear they had typhoid. All of them, it turned out, had been exposed to the pathogen on the same day. At a church luncheon and dinner on March 17, they had been served food made by a woman whom disease reports referred to as Mrs. X. Investigators discovered that she was a carrier for typhoid despite never, to her knowledge, having had symptoms. It was in some ways just another footnote in epidemiologists’ growing compendium of how asymptomatic carriers, like the notorious “Typhoid Mary” Mallon in early 20th-century New York, can spread illness, but it also posed an interesting question: If the townsfolk were all exposed to the pathogen at the exact same time, why was there such a difference in how long it took patients to feel sick?
That question has long percolated through the medical literature on the incubation time of diseases. It took on an extra wrinkle in 1950, when the epidemiologist Philip Sartwell reviewed the literature, including the report on the 1914 typhoid outbreak, and noted that the incubation times for many diseases, including polio, malaria and chicken pox, seemed to show similar patterns. They didn’t follow a normal distribution, the kind we call a bell curve, as would be expected if what caused the differences were regularly distributed across the population. Instead, the graphs usually had a long tail to the right, showing that many patients took a surprisingly long time to fall ill. Moreover, the distributions often appeared to be what’s called lognormal, which can happen, for example, when the numbers being plotted are the result of multiplicative processes rather than additive ones. It seemed like a weird commonality for diseases that, biologically, had almost nothing to do with one another.
On the face of it, the reason why some fall ill in a day while others take weeks seems likely to be connected to the differences in individuals’ immune systems. Often what starts the sensation of a scratchy throat or a drippy nose is the arsenal of molecules that the immune system makes to combat an invading pathogen rather than the pathogen itself. And we don’t all get the same dose of a pathogen: Touching a doorknob might expose you to far more or less than getting sneezed on. But these are qualitative explanations that don’t necessarily produce the observed distribution. If we understood why that happens, maybe we could get ahead of diseases.
Now a pair of mathematicians and a physician-scientist, interested in the dynamics of how sick cells take over networks of healthy ones, have discovered that a mathematical model of the process that relies on geometry and chance does yield just such a distribution. They didn’t start out by focusing on infections — their model began as a way to think about the spread of tumor cells — but they suspect it could inspire some new thinking about why this happens.
Applying their model to infections might be pushing it: Its details don’t fit well with the biology of many pathogens. On the other hand, their work highlights that current ideas about the distribution of incubation periods don’t always hold up well to mathematical scrutiny either.
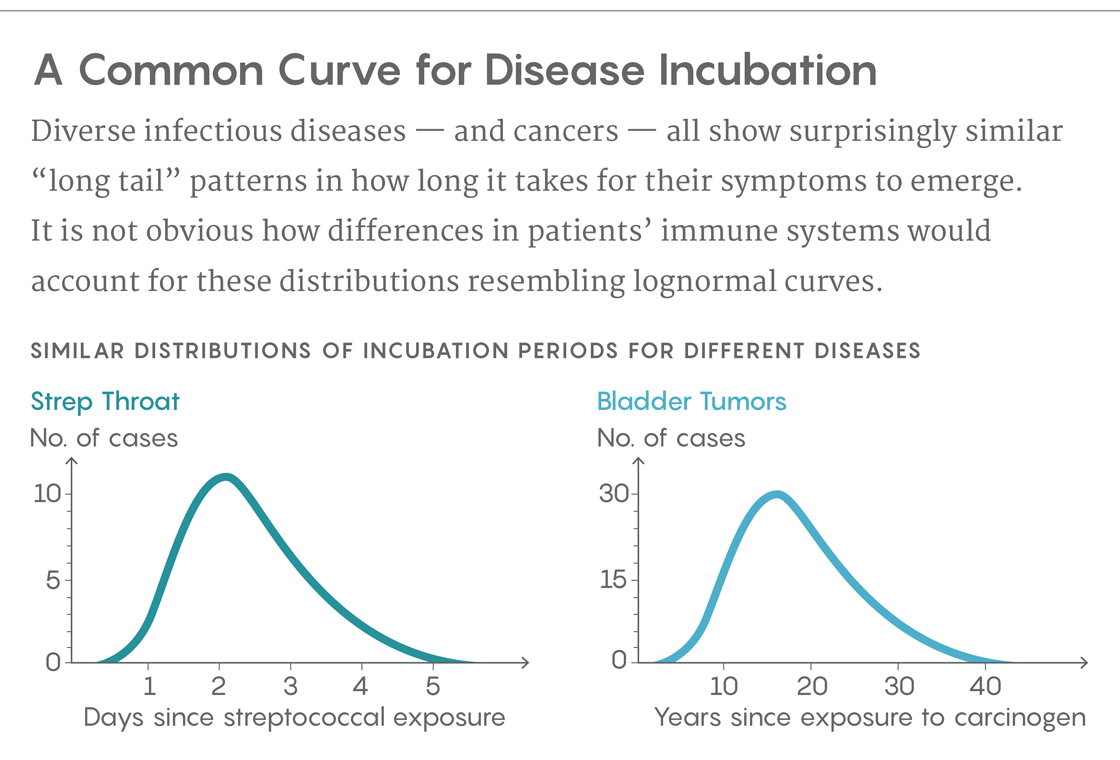
Lucy Reading-Ikkanda/Quanta Magazine; Source: DOI: 10.7554/eLife.30212
Jacob Scott, a radiation oncologist and mathematical biologist at the Cleveland Clinic, said that he and his two coauthors on the paper were initially considering how cancer spreads through a tissue. In experiments in which the trigger for a cancer’s development is known, the length of time for symptoms to appear falls along a lognormal distribution, rather than a bell curve. They wondered whether the geometry of tissue structures might hold a clue. “Studying tumors from a spatial perspective is really hard,” he said. “We’re just starting to scratch the surface of how to study that in a sensible way.” So they created a model to explore the question.
To understand their model, think of hundreds of cells packed together. Each cell touches its neighbors, and each of the neighbors touches its own neighbors and so on, forming a kind of network. When any cells die, one of their neighbors replicates itself to fill the gap.
Now imagine an invading cell arrives on the scene. As host cells die, neighboring host cells and invaders compete to fill the available openings with copies of themselves before the other can. How long it takes the invaders to conquer the whole network depends on how good they are at seizing real estate (and a few other parameters). If you run this scenario repeatedly and plot the varying times it takes to reach completion, you get a curve that closely resembles a lognormal distribution.
That distribution occurs for a couple of reasons. When the invaders are much stronger than the host cells — as in aggressive cancers, for instance — the invaders soon gain a healthy share of the territory but then run into a problem: Their only remaining neighbors are usually other invader cells, not hosts. With so few host cells left, the invaders’ spread slows dramatically. It can then take a paradoxically long time for them to root out the last host cell, generating that long skew to the right in the distribution.
Even if the host and invader cells are equally fit, and their competitions for space are decided randomly, the researchers saw that sometimes the invaders can eventually take over. And then, too, the times to completion fall along a curve that approximates a lognormal. The results of the researchers’ model suggest that this distribution can arise from something as simple and universal as the fact that cells exist in space with one another, and an invader must grapple with that arrangement as it spreads.
Partway through their project, the researchers began to realize that the cancer model they were developing might have more widespread relevance. When they then discovered Sartwell’s old paper on incubation times, the connection to infectious diseases clicked into place. Infectious organisms might not be replacing host cells, but their spread could be limited by the availability of uninfected cells in tissues. Disease symptoms might manifest only after the invaders took over a certain amount of a tissue network, and that time would vary between individuals along the curve Sartwell had observed.
“These disease processes are so different,” said Steven Strogatz, the Cornell University mathematician who coauthored the study with Scott and Bertrand Ottino-Loffler, a graduate student at Cornell. (Strogatz is also on the advisory board for Quanta.) Nevertheless, at an abstract, qualitative level, “the reason these look the same shape might be because they’re both about a network of healthy or normal cells being invaded.”
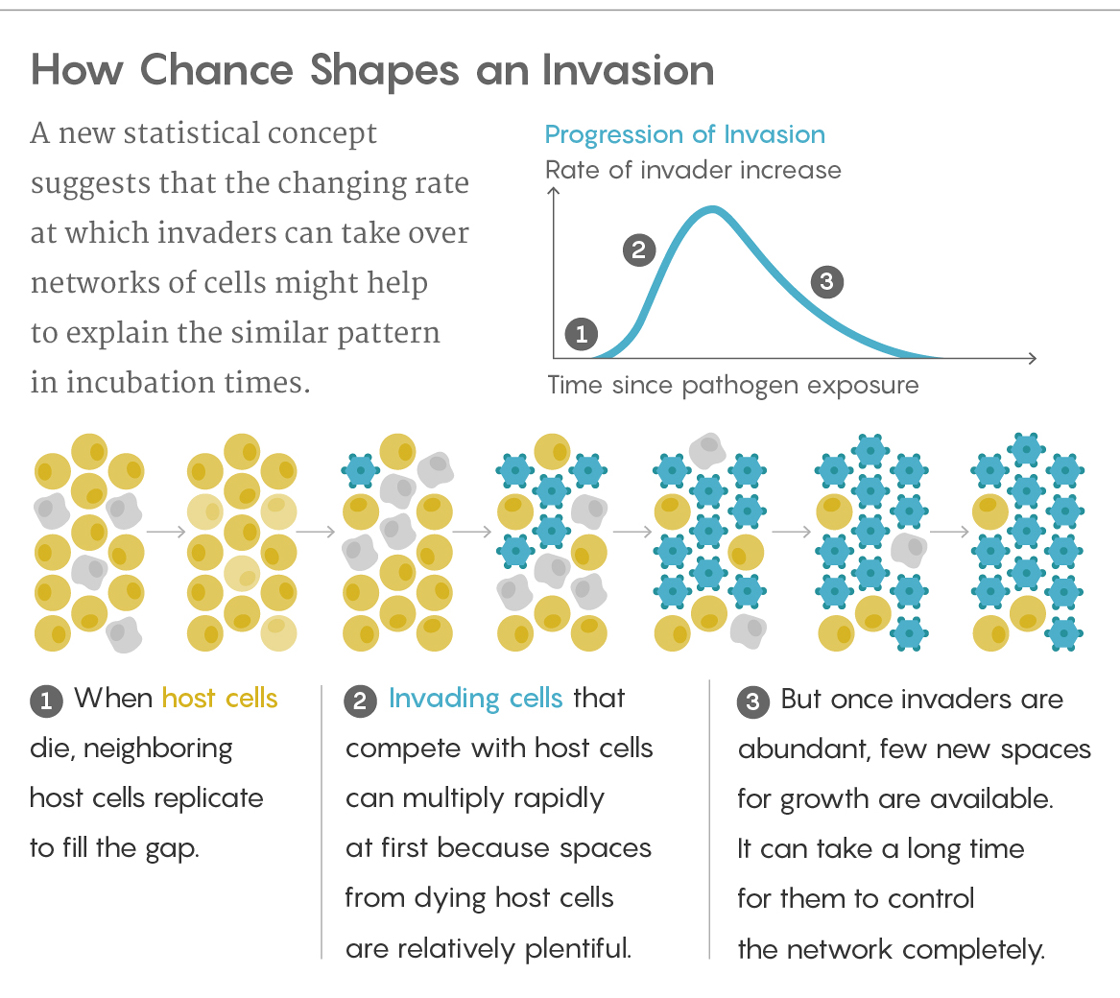
Lucy Reading-Ikkanda/Quanta Magazine
Biologists familiar with infectious disease, however, might point out that the specifics of this model don’t have easy correlates in the natural world. A process by which host cells have to die to make room for invaders is plausible for some cancers. It might make some sense in the context of a bacterial infection in the gut, where local microbial inhabitants are slaughtered or just outcompeted by invaders — or it might not. But as Scott, Strogatz and Ottino-Loffler acknowledge, it doesn’t reflect how viral infections often spread, with viruses building copies of themselves inside infected cells and then exploding outward to infect many neighbors at once.
That is disappointing because many viral infections do seem to have lognormal distributions for their incubation times, said Katia Koelle, a theoretical ecologist at Emory University who studies the population dynamics of viruses. “With these unsolved ubiquitous patterns, taking mathematical approaches is an incredibly interesting and worthwhile endeavor,” she says. However, the fact that this model does not look analogous to virus biology makes it unsatisfying.
The model’s authors readily admit its shortcomings when it comes to biological verisimilitude. But they also make the case that immunological explanations for infections’ varied incubation times would not lead to the distributions we see. “If you assume that the only variability in the host community is a normal distribution in the thresholds at which symptoms occur,” said Strogatz, meaning that people have different immune systems, “then such a model produces a left-skewed distribution. That prediction is contrary to what Sartwell saw.”
Indeed, many researchers cite Sartwell’s 1950 paper when they need to explain why they assume that a disease’s incubation time will show a lognormal distribution, according to Benjamin Althouse, a computational epidemiologist at the Institute for Disease Modeling in Bellevue, Washington. But in his view, no one seems to have done much to explain why that happens.
“It’s of fundamental importance to know why they are all lognormal,” he said. “If you know what’s driving the incubation period, you can invent interventions.”
Sam Scarpino, a computational epidemiologist at the Network Science Institute at Northeastern University who collaborates frequently with Althouse, added, “With influenza, for example, there is a pretty narrow window when we think you can deploy antivirals in order to have a measurable effect.” If that window varies quite a bit between individuals because of these incubation period effects, understanding why they happen will be crucial.
It’s possible that the diseases’ similar incubation distributions might have multiple explanations that coincidentally converge in effect. As Scott and his colleagues noted in their paper, if a pathogen grows exponentially and a population receives a statistically normal distribution of exposures to it, then a lognormal distribution of incubation times should result. The arrangement of cells in the tissues would be irrelevant.
To Aaron Clauset, a computer scientist at the University of Colorado who studies network dynamics, it would be interesting to know under what circumstances one of these outcomes is more likely and what other factors might be involved. “I like this style of research a lot because it reminds me of what made physics such a powerhouse in the 20th century — a division of labor between theoreticians and empiricists,” Clauset reflected. “I hope somebody will follow on the work and find out.”
For their part, Scott, Strogatz and Ottino-Loffler hope that others with more expertise in infectious diseases will see the paper and get curious about these curves. They published previous work on the subject in a physics journal. This time, they chose to submit to eLife, a well-regarded biology journal, in hopes that immunologists, epidemiologists and others would get interested in testing whether it fits with real-life data — or in coming up with a better explanation themselves.